Publications
Landscape of AI Legislation: Opportunities for Housing
This report features a joint collaboration between NFHA and the University of Michigan to comprehensively map the landscape of state level engagement on AI legislation in 2024 legislative sessions. The survey covers 480 bills across state jurisdictions to identify current macro level trends across both general AI use cases and sector specific insight on AI activities in the housing sector. Findings highlight that legislators have an increased interest in regulating the use of AI for dynamic rental pricing, suggesting a growing sensitivity to the downstream effects of AI on consumer access. Similarly, the majority of AI bills introduced sought to bolster existing consumer protections in light of the distinct utility and harm potential that AI systems bring to consumer-oriented application contexts. This project was conducted under STPP’s Community Partnerships Initiative which is funded by the Ford Foundation.
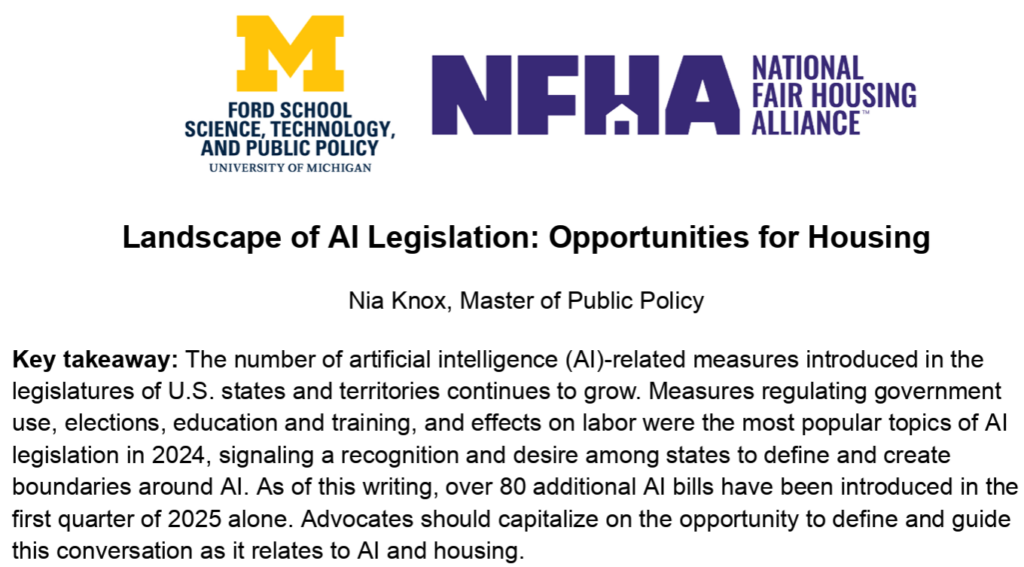
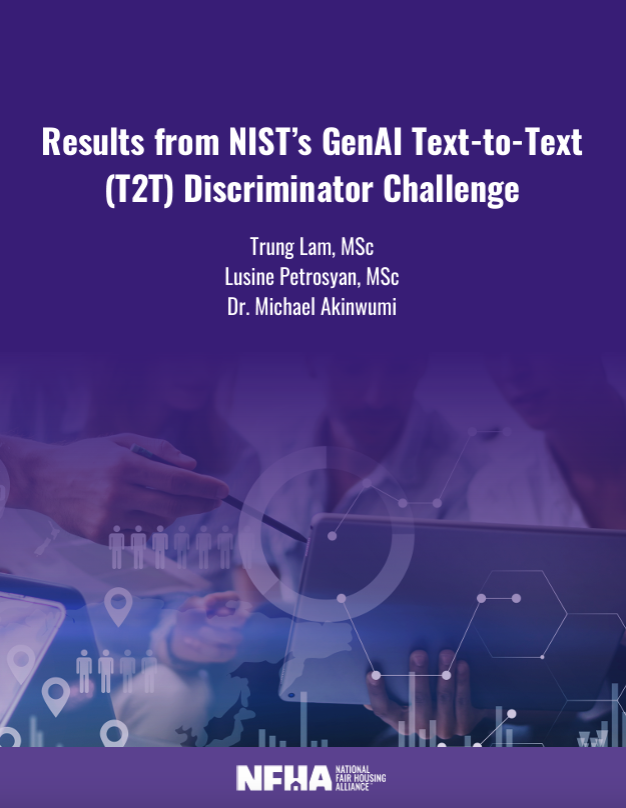
Results from NIST’s GenAI Text-to-Text (T2T) Discriminator Challenge
This report details findings from NFHA’s participation in National Institute of Standards and Technolgy’s GenAI Text-to-Text (T2T) Discriminator Challenge, which aimed to develop methods for distinguishing between AI-generated and human-generated text. The study was motivated by concerns about AI’s increasing role in housing and real estate, particularly regarding potential discrimination and bias. The authors tested various machine learning models, with Support Vector Machine (SVM) and XGBoost showing the most promising results in differentiating between AI and human-generated content.
The findings highlight both opportunities and challenges in AI content provenance. While some models achieved high accuracy rates, identifying specific AI platforms (like ChatGPT, Claude, or Gemini) proved more difficult. The report outlines five key policy implications: platform policy and content moderation, transparency and accountability, auditing and detection tools, literacy and awareness, and high-risk use cases. The research underscores the importance of developing robust detection methods to ensure fair housing practices and prevent discriminatory AI-generated content, while acknowledging the need for continued research to improve source attribution accuracy and reliability.
From Policy to Practice: Essential Competencies for Federal Chief AI Officers
The report explores the crucial role of Chief AI Officers (CAIOs) in ensuring the responsible and effective use of artificial intelligence (AI) within federal agencies. It emphasizes that the increasing use of AI in government necessitates proper governance and risk management. It highlights that the success of AI initiatives hinges on the selection of suitable CAIOs who possess the necessary skills and expertise to navigate the complexities of AI implementation and oversight.
The report also underscores the importance of transparency from agencies regarding their AI governance efforts and the qualifications of their CAIOs. The research conducted by the National Fair Housing Alliance (NFHA) examines the alignment between the experience of appointed CAIOs and the requirements of their roles, offering insights into the qualifications essential for effective AI leadership. It concludes by proposing strategies for CAIOs to fulfill their responsibilities and considerations for enhancing agency accountability and transparency to the public.
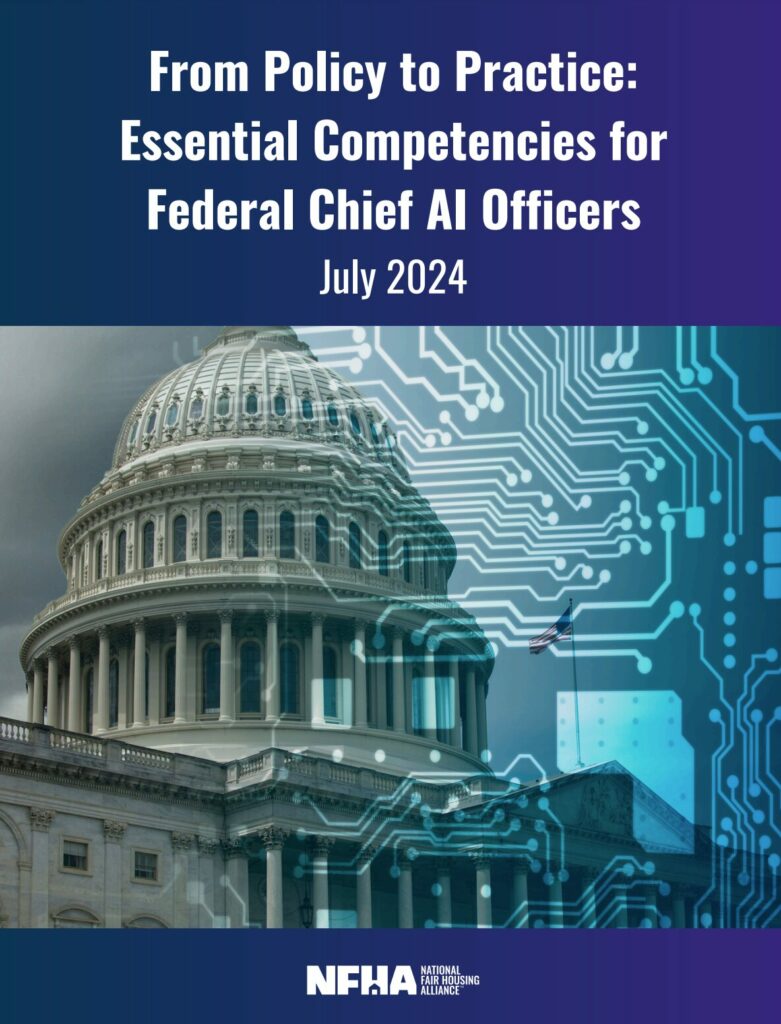
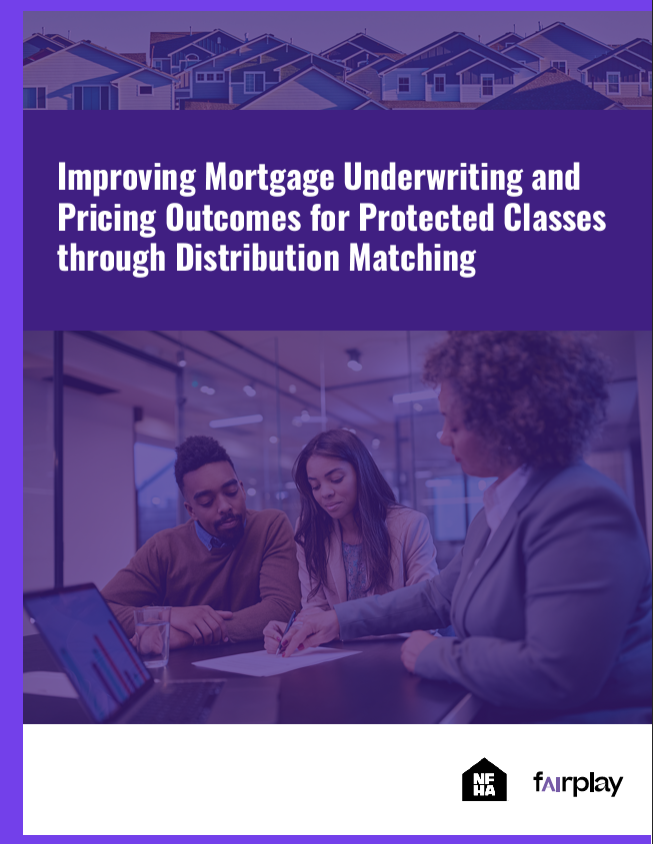
Unlocking Fairness: Improving Mortgage Underwriting and Pricing Outcomes for Protected Classes through Distribution Matching
The report presents the preliminary findings of a joint study by the National Fair Housing Alliance (NFHA) and FairPlay AI, which investigates the potential of AI and machine learning to improve fairness in mortgage underwriting and pricing, particularly for historically underserved groups. Using a novel methodology called Distribution Matching (DM), the study finds that it is possible to reduce disparities for protected groups, such as Black and Hispanic borrowers, by up to 13% in underwriting and up to 20% in pricing, without losing model accuracy.
The preliminary findings suggest that DM can significantly reduce disparities in mortgage underwriting and pricing outcomes for Black and Hispanic borrowers compared to White, non-Hispanic borrowers, without compromising model accuracy. The study also highlights the limitations of the available data and recommends further research with an enriched dataset to validate and expand upon these findings. Overall, the study offers a promising approach to addressing persistent discrimination in mortgage lending through the use of AI and algorithmic fairness techniques.
Privacy, Technology, and Fair Housing – A Case for Corporate and Regulatory Action
This report, co-authored by the National Fair Housing Association (NFHA) and TechEquity Collaborative, examines the intersection of privacy, technology, and fair housing. It emphasizes the need for a balanced approach to privacy and civil rights in the housing sector, especially as algorithms and AI systems increasingly influence decisions in mortgage lending, tenant screening, and other areas. The report advocates for stronger regulatory frameworks, data minimization practices, and privacy-preserving technologies to protect consumers while ensuring non-discriminatory access to housing.
The report emphasizes three essential shifts to ensure future protection in the context of AI and housing: first, enhancing the review and approval process for AI tools prior to their deployment; second, shifting the responsibility for addressing harm from individuals to corporations and regulatory bodies; and third, incorporating an intersectional approach in the design and regulation of AI tools and models. The report also discusses the challenges of enforcing civil rights in the digital era and suggests integrating privacy with civil rights protections to prevent discrimination and promote fairness in housing.
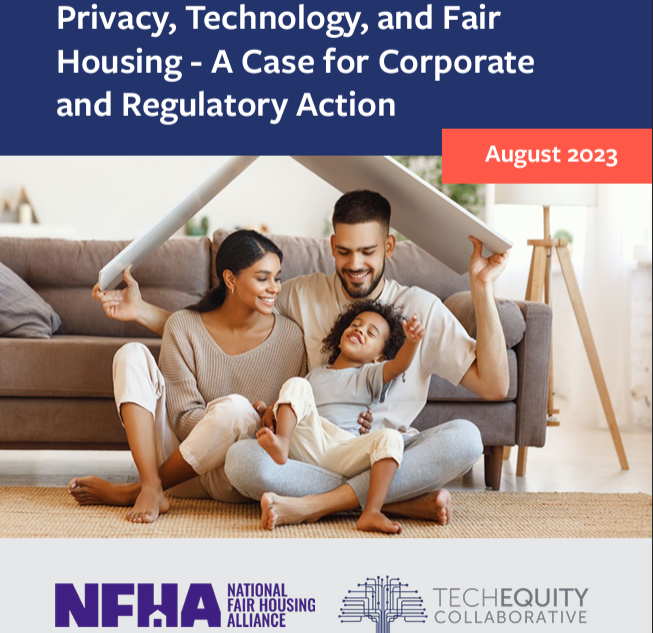
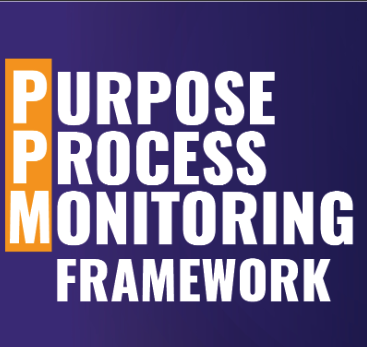
Purpose, Process, and Monitoring (PPM) Framework for Auditing Algorithmic Bias in Housing & Lending
PPM is a new framework for comprehensively auditing algorithmic systems in sectors like housing and lending. The framework aims to address the potential for algorithmic bias and discrimination, which can lead to severe consequences for individuals and perpetuate existing inequities.
The first stage, Purpose, examines the goals of the algorithmic system, the business problem it seeks to solve, and how data is used to represent the problem. It emphasizes the importance of data representativeness and mitigating risks associated with data limitations. The second stage, Process, evaluates the design and development of the algorithmic solution, including the team’s composition, data assessment, model assessment, outcome assessment, and model use and limitations. It stresses the need for diverse and well-trained teams, thorough data analysis, and careful model selection and validation. The final stage, Monitoring, focuses on the ongoing monitoring of the model in the production environment to ensure its continued validity, robustness, and fairness. It includes production model validation and protection from confidentiality and integrity attacks.
The PPM framework offers a holistic approach to auditing algorithmic systems, going beyond traditional fair lending analysis. It enables auditors to identify assumptions and limitations in the system and provide recommendations to mitigate consumer fairness and privacy risks. The framework draws upon existing resources like the Model Risk Management guidance, the NIST proposal for identifying and managing bias in AI, and the CRISP-DM process. The ultimate goal of the PPM framework is to promote fairness, accountability, transparency, explainability, and interpretability in algorithmic systems, particularly in critical sectors like housing and lending.
An AI Fair Lending Policy Agenda for the Federal Financial Regulators
Authors discuss the increasing use of artificial intelligence and machine learning (AI/ML) in consumer finance and the potential for these technologies to perpetuate discrimination against historically underserved groups. The authors argue that while AI/ML offers benefits such as increased efficiency and accuracy, it can also amplify existing biases in the data, leading to disparate impact on protected classes. The paper proposes a policy agenda for federal financial regulators to mitigate these risks, including setting clear expectations for fair lending testing, clarifying the search for less discriminatory alternatives, broadening Model Risk Management guidance, providing guidance on third-party models, and improving data transparency and research. The authors emphasize the importance of regulatory action to ensure that AI/ML is used to promote financial inclusion and fairness, rather than exacerbating existing inequalities.
These measures aim to ensure that AI/ML systems used in credit decisions do not reinforce existing biases and discrimination, and instead, contribute to more equitable access to financial services.
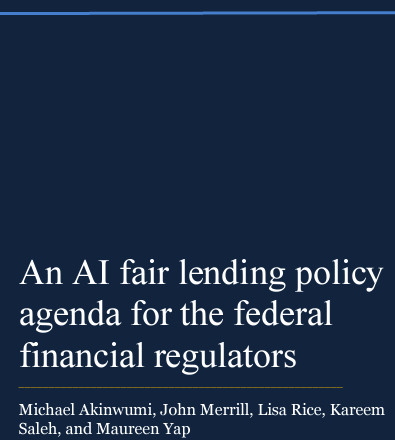